subsample(Subsampling A Better Way to Analyze Data)
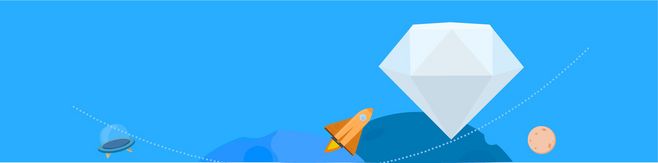
Subsampling: A Better Way to Analyze Data
Data analysis is an essential aspect of modern-day research and development. It is a process of extracting meaningful insights from large sets of data. However, the analysis of such large data sets can be overwhelming and time-consuming. One solution to this problem is subsampling, where a smaller sample of data is taken from the larger dataset for analysis.
What is subsampling?
Subsampling, as the name suggests, is the process of taking a smaller sample from a larger dataset. The sample is chosen randomly, but it contains a representative portion of the entire dataset. Subsampling is commonly used in statistical analysis, machine learning, and data science for various purposes.
The purpose of subsampling is to reduce the computational time and make the analysis more manageable. It can also help in reducing overfitting and generalizing the model. Subsampling can be done in two ways, simple random sampling and stratified sampling.
Simple Random Sampling
Simple random sampling is the most basic and straightforward way of subsampling. In this method, a random sample is taken from the entire dataset, and all the data points have an equal chance of being selected. Simple random sampling is the best method for homogenous datasets, where all the data points have a similar distribution.
However, simple random sampling may not be the best method for datasets that have a skewed distribution. In such cases, stratified sampling may be more suitable.
Stratified Sampling
Stratified sampling is a more complex way of subsampling, but it can be more useful in certain cases. A dataset can be divided into several strata based on a specific criteria. The data in each stratum is similar, but it may differ from the data in other strata. In stratified sampling, a random sample is selected from each stratum according to its proportion in the entire dataset.
Stratified sampling is especially useful when the dataset has a skewed distribution or when there is a limited number of samples in a specific category. In such cases, stratified sampling can ensure that each category is well-represented in the sample.
In conclusion
Subsampling can be a powerful tool for data analysis, and it is widely used in various fields. The choice of method depends on the nature of the dataset and the research question. Simple random sampling is the best method for homogenous datasets, while stratified sampling is more useful for skewed datasets. Regardless of the method chosen, subsampling can save time, reduce computational costs, and improve the accuracy of the analysis.