coefficient(Understanding the Coefficient A Key Metric for Data Analysis)
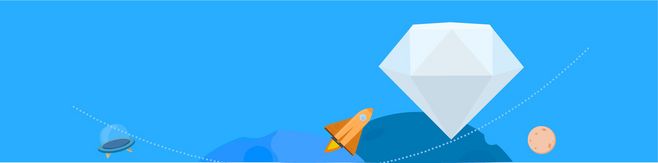
Understanding the Coefficient: A Key Metric for Data Analysis
Introduction:
Data analysis plays a crucial role in modern businesses, with decision-making heavily relying on the insights derived from data. Coefficients are a fundamental metric used in data analysis to understand the relationships and trends within datasets. In this article, we will explore the concept of coefficients, their significance, and how they are calculated.
1. What are Coefficients?
Coefficients are numerical values that quantify the strength and direction of relationships between variables in a dataset. These variables can be anything from sales figures, customer demographics, to employee performance. Coefficients help to determine how one variable affects another, providing valuable insights into patterns and trends.
2. Types of Coefficients:
There are various types of coefficients, each serving a different purpose in data analysis. Some of the most commonly used coefficients include:
a. Pearson's Correlation Coefficient:
Pearson's Correlation Coefficient is used to measure the linear relationship between two continuous variables. It determines the strength and direction of the relationship through a value ranging from -1 to 1. A coefficient close to 1 indicates a strong positive relationship, while a coefficient close to -1 indicates a strong negative relationship. A coefficient of 0 implies no relationship.
b. Coefficient of Determination (R-squared):
The coefficient of determination, commonly known as R-squared, measures the proportion of the variance in the dependent variable that is predictable from the independent variable(s). R-squared ranges from 0 to 1, with a higher value indicating a stronger relationship between the variables.
c. Beta Coefficient:
The beta coefficient measures the sensitivity of the dependent variable to changes in the independent variable. It is widely used in the field of finance to assess the risk associated with an investment. A beta coefficient greater than 1 indicates higher volatility than the market, while a coefficient less than 1 suggests lower volatility.
3. Calculating Coefficients:
To calculate coefficients, statistical methods and formulas are applied to the dataset. The specific calculation depends on the type of coefficient being calculated. For instance, calculating Pearson's Correlation Coefficient involves determining the covariance and standard deviations of the variables under consideration. Likewise, calculating R-squared involves fitting a regression model to the data.
Conclusion:
Coefficients are essential metrics used in data analysis to quantify relationships and understand trends within datasets. Pearson's Correlation Coefficient, Coefficient of Determination (R-squared), and Beta Coefficient are just a few examples of the wide variety of coefficients available for different types of analysis. By calculating and interpreting these coefficients, businesses can make informed decisions based on data-driven insights, enabling them to optimize their operations and achieve their goals.
Understanding the significance of coefficients is crucial in harnessing the power of data analysis and deriving meaningful insights for business growth. Incorporating coefficients in data analysis strategies empowers organizations to make informed decisions, predict trends, and take appropriate actions, ultimately leading to their success in a highly competitive market.