subbranch(Exploring the Subbranch of Artificial Intelligence Natural Language Processing)
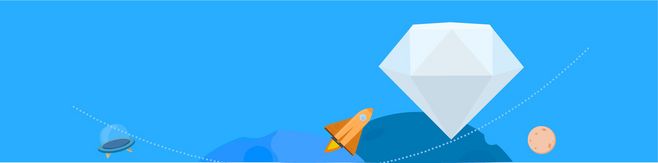
Exploring the Subbranch of Artificial Intelligence: Natural Language Processing
Natural Language Processing (NLP) is a prominent subbranch of Artificial Intelligence (AI) that focuses on the interaction between computers and human language. It involves the development of algorithms and models to enable machines to understand, interpret, and respond to human language in a way that is both meaningful and useful. In this article, we will delve into the fascinating world of Natural Language Processing, exploring its applications, advancements, and challenges.
The Applications of NLP
NLP has found numerous applications across various industries, transforming the way we interact with computers and machines. One of the most common applications of NLP is machine translation. With the advancement of NLP algorithms, machines are now able to automatically translate text from one language to another, making it easier for people to communicate and access information across different languages. Moreover, NLP has also played a crucial role in the development of virtual assistants such as Siri and Alexa, enabling users to interact with their devices through natural language commands and queries.
Another important application of NLP is sentiment analysis. By analyzing social media posts, customer reviews, and other texts, NLP algorithms can determine the sentiment or emotion behind the text. This information is valuable to companies as it allows them to understand customer feedback and opinions on their products or services. NLP-powered sentiment analysis can also be used to identify and monitor public sentiment towards political candidates, brands, or social issues, helping businesses and governments make informed decisions.
Advancements in NLP
Over the years, NLP has witnessed significant advancements, thanks to the availability of large amounts of data and the development of powerful machine learning algorithms. One major breakthrough in NLP is the introduction of deep learning models, such as recurrent neural networks (RNNs) and transformers. These models have revolutionized the field by enabling machines to process and understand language more effectively. For example, the Transformer model, introduced by Google in 2017, has achieved state-of-the-art performance in various natural language processing tasks, including machine translation and text summarization.
Another notable advancement in NLP is the pre-training and fine-tuning approach. This approach involves training a language model on a large corpus of data and then fine-tuning it on a specific task. This method has shown impressive results in various NLP tasks, including question-answering, document classification, and named entity recognition. The pre-trained models, such as BERT (Bidirectional Encoder Representations from Transformers), have become the cornerstone of many NLP applications, providing a strong foundation for further research and development in the field.
Challenges in NLP
Despite the remarkable progress in NLP, several challenges still exist. One major challenge is the ambiguity and complexity of human language. Language is context-dependent and often relies on implicit knowledge and understanding. NLP models often struggle to accurately capture the nuances and subtleties of human language, leading to errors and misunderstandings. The development of more sophisticated models and algorithms that can better handle ambiguity and context is an ongoing area of research in NLP.
Another challenge in NLP is the lack of training data for certain languages and domains. NLP models heavily rely on large amounts of labeled data for training, but for many languages and specific domains, such data may be limited or nonexistent. This poses challenges for researchers and developers working on NLP applications that require data in these domains. Efforts are being made to collect and create more diverse and comprehensive datasets to address this challenge in NLP.
In conclusion, Natural Language Processing has emerged as a critical subbranch of Artificial Intelligence, with a wide range of applications and advancements. From machine translation to sentiment analysis, NLP has transformed the way we communicate and interact with machines. While significant progress has been made, challenges still exist in accurately understanding and interpreting human language. However, with ongoing research and technological advancements, we can expect NLP to continue pushing boundaries and unlocking new possibilities in the future.